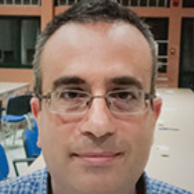
In 1948 the famous MIT professor Norbert Wiener published his book Cybernetics or Control and Communication in the Animal and the Machine. The term cybernetics comes from the ancient Greek word kybernetikos (good at steering), referring to the art of the helmsman. Cybernetics is interdisciplinary in nature. Based on common relationships between humans and machines, it is used in control and automation theory to reduce many time-consuming computations and decision-making processes, formerly done by human beings.
Cybernetics can be considered as the foundation of modern decision-making. The availability of massive amounts of data, improvements in analytic methodologies, and substantial increases in computing power, have resulted in a significant upsurge in the use of data-driven decision making. We talk about operations research, decision support systems, business analytics, business intelligence, data science, machine learning and so on. So, what are the differences between all these disciplines? Let’s take a closer look.
The roots of operations research (OR) can be traced back many decades, when early attempts were made to use a scientific approach in the management of organizations. The official beginning of the activity has generally been attributed to the military services in World War II. Because of the war, there was an urgent need to allocate scarce resources to various military operations in an effective manner. Therefore, the British and the US military management called upon a large number of scientists to apply a scientific approach to dealing with strategic and tactical problems. In effect, they were asked to do research on (military) operations (Hillier & Liebermann, 2015).
When the war ended, the success of OR spurred interest in non-military applications. As the industrial boom following the war was running its course, the problems caused by the increasing complexity and specialization in organizations, came to the forefront. It was becoming apparent to business consultants who had served with the OR teams during the war, that these were basically the same problems that had been faced by the military, but in a different context (Hillier & Liebermann, 2015). By the early 1950s, these individuals had introduced the use of OR to a variety of organizations in business, industry, and government. The rapid spread of OR soon followed.
OR is applied to problems that concern how to conduct the activities within an organization. The nature of the organization is essentially immaterial, and in fact, OR has been applied extensively in such diverse areas as manufacturing, transportation, construction, telecommunications, financial planning, health care, and public services. Therefore, the breadth of application is unusually wide. It is evident that no single individual should be expected to be an expert on all the many aspects of OR. This would require a group of individuals having diverse backgrounds and skills. Such an OR team typically needs to include people who are highly trained in statistics, economics, business administration, computer science, and engineering (Hillier & Liebermann, 2015).
Analytics (or business analytics) is considered as the successor of OR. There has been great buzz throughout the business world in recent years about the importance of incorporating analytics into managerial decision making. The primary impetus for this buzz is associated with Thomas Davenport, a renowned thought-leader who has helped hundreds of companies worldwide to revitalize their business practices (Davenport & Harris, 2007). So what is analytics? The short (but oversimplified) answer is that it is basically OR by another name (Hillier & Liebermann, 2015). However, there are differences in their relative emphases. Analytics fully recognizes that we have entered into the era of big data, where massive amounts of data now are available to many businesses and organizations to support managerial decision making.
As indicated by the following definition, a primary focus of analytics is on how to make the most effective use of all these data: Analytics is the scientific process of transforming data into insight for making better decisions (definition developed by the Institute for Operations Research & the Management Sciences, INFORMS). It has been stressed that business analytics are tightly connected with big data, i.e., any set of data that is too large or too complex to be handled by standard data-processing techniques and typical desktop software (Camm et al., 2019). IBM describes the phenomenon of big data through the four Vs: volume (data at rest), velocity (data in motion), variety (data in many forms), and veracity (data in doubt).
The application of analytics can be divided into three overlapping categories. The first, descriptive (or reporting) analytics, encompasses innovative techniques to locate the relevant data and identify the interesting patterns to better describe and understand what has happened in the past and what is going on now. Examples are data queries, descriptive statistics, data visualization, dashboards, data mining techniques, and fundamental if-then spreadsheets. A second (and more advanced) category is predictive analytics, which involves using the data to predict what will happen in the future or ascertain the impact of one variable on another. Regression analysis, time series, and statistical forecasting are prominently used here. The final (and most advanced) category is prescriptive analytics, which involves using the data to prescribe what should be done in the future. More specifically, prescriptive analytics indicates a course of action to take. Thus, the output of a prescriptive model is a decision. The powerful optimization techniques of OR generally are what are utilized here, along with simulation, expert systems, decision modeling etc.
According to Hillier & Liebermann (2015), OR analysts often deal with all three of these categories, but not very much with the first one, somewhat more with the second one, and heavily with the last one. Thus, OR can be thought of as focusing mainly on advanced analytics, predictive and prescriptive activities, whereas analytics professionals might get more involved than OR analysts with the entire business process, including what is associated with the first category (identifying a need) and what follows the last category (implementation). Looking to the future, the two approaches should tend to merge over time. Because the term analytics is more meaningful to most people than the term OR, it is concluded that analytics may eventually replace OR, as the common name for this integrated discipline.
Although analytics was initially introduced as a key tool mainly for business organizations, it also can be a powerful tool in other contexts. As one example, analytics, together with OR, played a key role in the 2012 presidential campaign in the US (Hillier & Liebermann, 2015). The Obama campaign management hired a multi-disciplinary team of statisticians, predictive modelers, data-mining experts, mathematicians, software programmers, and OR analysts. It eventually built an entire analytics department five times as large as that of its 2008 campaign. With all this analytics input, the Obama team launched a full-scale and all-front campaign, leveraging massive amounts of data from various sources to directly micro-target potential voters and donors with tailored messages.
However, apart from OR and business analytics, machine learning (ML) constitutes another immense element of the underlying discussion. ML is a branch of artificial intelligence (AI). AI is concerned with developing ways in which machines can imitate human intelligence, possibly improving on it. ML involves the creation of intelligence by learning from large volumes of data. This is critical, considering that data available for ML is growing exponentially. It is estimated that in any two-year period we generate nine times as much data as existed at the beginning of the two years (Hull, 2020).
There are four main categories of ML models (Hull, 2020). First, supervised learning is concerned with using data to make predictions. Second, unsupervised learning is concerned with recognizing patterns in data. The main objective is not to forecast a particular variable. Rather it is to understand the environment represented by the data better. Third, semi-supervised learning is a hybrid between supervised and unsupervised learning. It arises when we are trying to predict something and we have some data with labels and some unlabeled data. And fourth, reinforcement learning, is concerned with situations where a series of decisions is to be taken. In those circumstances the environment is typically changing in an uncertain way, as the decisions are being taken.
Looking back to history, it was in the 1970s that the primary focus of information systems for decision making focused on providing structured, periodic reports that a manager could use for decision making (Sharda et al. 2018). Businesses began to build decision support systems (DSSs) and create routine reports to inform decision makers about what had happened in the previous period. In the 2000’ DSSs began to be called business intelligence (BI) systems. BI is an umbrella term that combines architectures, tools, data-bases, analytical tools, applications, and methodologies.
Even as the concept of BI and analytics is receiving more attention in industry and academic circles, another term has already been introduced and is becoming tremendously popular. The new term is data science (Bowne-Anderson, 2018). The distinction between analytics and data scientist is more of a degree of technical knowledge and disciplines, than functions. Computer science, statistics, and applied mathematics academic programs appear to prefer the data science label, reserving the analytics label for more business-oriented professionals (Sharda et al. 2018).
References
Bowne-Anderson, H.: What Data Scientists Really Do, According to 35 Data Scientists, Harvard Business Review, August 2018.
Camm, J. D., J. J. Cochran, M. J., Fry, J. W. Ohlmann, D. R. Anderson, D. J., Sweeney, and T. A. Williams: Business Analytics (3rd Edition), Cengage, Boston, MA, 2019.
Davenport, T. H., and J. G. Harris: Competing on Analytics: The New Science of Winning, Harvard Business School Press, Cambridge, MA, 2007.
Hillier, F. S., and G. J. Lieberman: Introduction to Operations Research (10th edition), McGraw-Hill, New York, 2015.
Hull, J.: Machine Learning in Business: An Introduction to the World of Data Science (2nd Edition), 2020.
Sharda, R., D. Delen, E. Turban: Business Intelligence, Analytics & Data Science: A Managerial Perspective (4th Edition), Pearson, 2018.
Wiener, N., 1948. Cybernetics: Or Control & Communication in the Animal & the Machine. 2nd Edition, 2019 reissue, Cambridge, MA: The MIT Press.